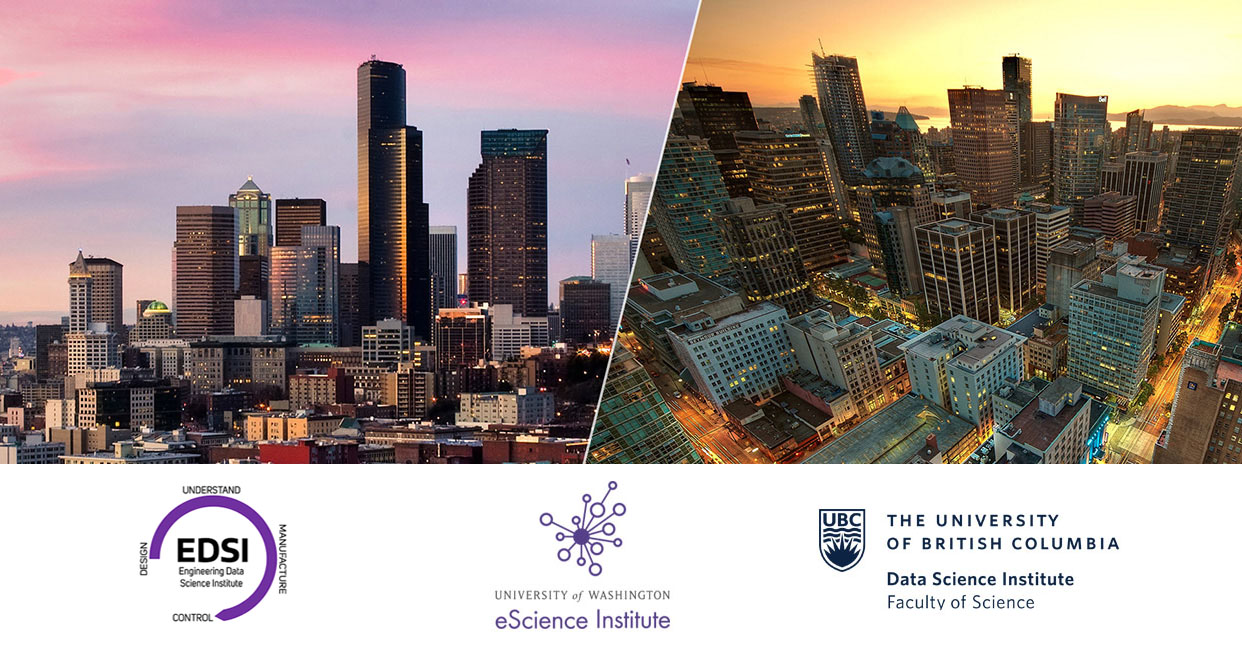
Northwest Data Science Seminar Series
The 2020 Northwest Data Science Seminar Series (NDSSS) features early career data science researchers from the University of Washington and University of British Columbia. These rising stars will present some of their latest research through online seminars and answer your questions in 20 minute Q&A sessions. This series is organized by the eScience Institute at UW and the Data Science Institute at UBC.
Registration for the talks is required. Please complete the RSVP form to receive details on how to join the webinars.
REGISTER FOR EVENT
Calendar of Speakers (all talks from 3PM – 4PM PST):
July 22 (Theme: Sensors and Data)
![]() |
Catherine Kuhn (Environmental Sciences, UW) No evidence of arctic-boreal lake greening Arctic and boreal ecosystems hold the highest concentration of the world’s lakes and are also undergoing the most rapid warming. Lake color is a designated Essential Climate Variable, but so far few studies have investigated changes in lake color at the global scale. Many global studies have used satellite observations to identify greening and browning trends associated with vegetation and carbon cycle dynamics in terrestrial ecosystems, yet changes in lake surface color have yet to be established at pan-arctic scales. Here we present decadal trends in arctic and boreal lake color derived using the high-resolution Landsat archive. We calculated annual growing season lake color from 1984 – 2019 for ~400,000 lakes and discovered overall declines in lake greenness over this 35-year period. Using ERA5 climate reanalysis data, we show that declines in lake greenness are 2.5 times greater in areas with enhanced warming and precipitation. In high northern latitudes, warmer and wetter conditions increase connectivity between lakes and the land surface, resulting in browning trends that are more apparent over continuous permafrost regions. In certain regions, however, lakes are greening. The observed shifts suggest that lake color of arctic and boreal lakes is undergoing significant changes in a warming climate. Link to recorded talk: https://youtu.be/-JfrpoLqh5c |
![]() |
Trevor Campbell (Statistics, UBC) Sparse Variational Inference: Bayesian Coresets from Scratch Abstract: Automated inference algorithms in Bayesian statistics have provided practitioners newfound access to fast, reproducible data analysis. But designing automated methods that are also computationally scalable and theoretically sound remains a significant challenge. Bayesian coresets takes the approach of compressing the dataset before running inference, providing scalability and guarantees on posterior approximation error. But the automation of past coreset methods is limited; they depend on the availability of a coarse posterior approximation, which is difficult to specify. In the present work we remove this requirement by formulating coreset construction as sparsity-constrained variational inference. This perspective leads to a novel construction via greedy optimization, and also provides a unifying information-geometric view of coreset methods. The proposed coreset construction algorithm is fully automated, requiring no problem-specific inputs aside from the probabilistic model and dataset. In addition to being significantly easier to use than past methods, experiments demonstrate that the proposed algorithm provides state-of-the-art Bayesian coreset constructions. Link to recorded talk: https://youtu.be/_8caIFEeBhY |
July 29 (Theme: Data and Privacy)
![]() |
Lalit Jain (Business, UW) Applications of Adaptive Experimenation Abstract: Scientific discovery is driven by a researchers ability to collect high quality data relevant to either verifying or disproving a hypothesis as quickly as possible. In recent years, a paradigm addressing this problem known as adaptive experimental design (AED) has been gaining traction. AED uses past measurements to inform the researcher what future measurements they should collect in a closed loop. In practice, AED has the potential to guide researchers to a conclusion with far fewer samples than any fixed data collection scheme. In this talk, we discuss some recent AED methods for best arm identification, and multiple hypothesis testing. We will also discuss a variety of applications including deciding the best caption for a cartoon, choosing amino acids sequences that form stable proteins, and running thousands of A/B tests on a large scale web platform. Link to recorded talk: https://youtu.be/uee_DmlMS6c |
![]() |
Mathias Lécuyer (Computer Science, UBC) Towards a Practical Differentially Private Machine Learning Platform Abstract: Companies increasingly expose machine learning (ML) models trained over sensitive user data to untrusted domains, such as end-user devices and wide-access model stores. Controlling the data’s leakage through these models is a major concern, both to meet users’ expectations, to enable broader ML use-cases, and to fulfill recent regulatory requirements. Despite this urgent need, most existing privacy work focuses on specific algorithms in isolation. They do not handle the complex requirements of ML platforms that serve entire workloads of models, which are constantly updated on an endless stream of data.
In this talk, I will present an ongoing effort to enable end-to-end differential privacy (DP) in ML platforms, and bound the cumulative leakage of training data through all models managed by the platform. I will focus on a recent proposal that contributes pragmatic solutions to two of the most pressing practical challenges of global DP: running out of privacy budget, and the privacy-utility tradeoff. I will then expand on new system design opportunities that this proposal opens, to further support DP in ML platforms. Link to recorded talk: https://youtu.be/4aDVC2y_wWs |
August 12 (Theme: Statistical and Machine Learning)
![]() |
Amy Willis (Biostatistics, UW) Paradoxes arising from model misspecification: a case study in microbiome data analysis Abstract: A microbiome is a collection of microscopic organisms that inhabit an environment. The human microbiome plays an important role in many human diseases, including diabetes, obesity, inflammatory bowel disease, asthma, and sexually transmitted diseases. Microbial communities are typically observed indirectly through high-throughput sequencing, and the number of times each strain is observed in a sample can be counted. We show empirical evidence that the observed fraction of genomic sequences from a bacterial strain is a biased estimate of its relative abundance. This observation is in direct conflict with most statistical models for microbial abundances. Furthermore, it can lead to counterintuitive conclusions about the direction of changes in abundances. We present this as a case study of the dangers of model misspecification, and as an invitation to quantitative researchers to validate their model’s assumptions prior to methods development. This research is joint work with David Clausen (UW), Michael McLaren (NCSU) and Ben Callahan (NCSU). Link to recorded talk: https://youtu.be/HXG39jIS_kw |
![]() |
Ben Bloem-Reddy (Statistics, UBC) Title: Bayesian inference for evolving networks with unobserved history Abstract: Many popular probability models for networks posit a network that evolves over time. Despite those models’ ability to reproduce certain observed phenomena, they have been of limited use as statistical models for networks with unobserved history. Examples include various biological networks or off-line social networks. I will discuss challenges and (partial) solutions to the inference problem in the context of two particular probability models: the ubiquitous Preferential Attachment model, and a generalization that creates new edges based on random walks. Link to recorded talk: https://youtu.be/9l9zsx_tpwk |
Planning Committee:
- Sarah Stone, eScience Institute UW
- David Beck, eScience Institute UW
- Jane Koh, eScience Institute UW
- Raymond Ng, Data Science Institute UBC
- Kevin Lin, Data Science Institute UBC